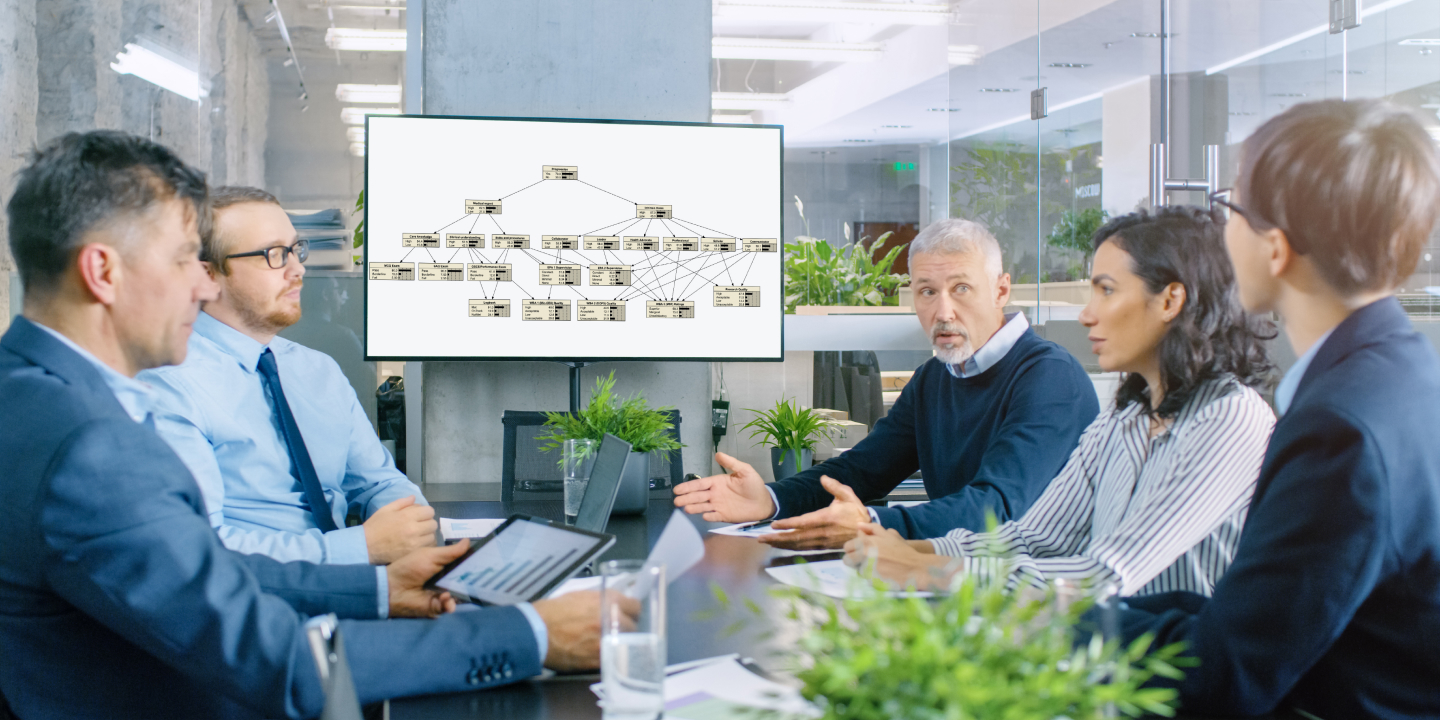
Supporting judgements with statistical modelling
Research 15 Feb 2021 8 minute readBayesian Networks can aid committees in managing evidence accumulation, help them make inferences under conditions of uncertainty, and buttress decisions by adding a layer of defensibility to the programmatic assessment process.
Medical colleges around the world are embracing holistic approaches to assessment that focus progression decisions on overall performance against different domains or proficiencies, rather than performance on high-stakes examinations alone.
‘Programmatic assessment’ is one such approach in which high-stakes decisions regarding trainee progression are made based on the accumulation of multiple lower-stakes data points, such as work-based assessments, logbooks, supervisor feedback and other relevant tasks, alongside exams.
Find out more:
Nathan Zoanetti is presenting at our fully online Research Conference 2021. Register now!
The programmatic approach champions the considered input of suitably qualified committees of experts to marshal these rich data into an evidence-based argument about whether the trainee is ready to progress. With this kind of approach, it is difficult to ensure that consistent judgements are being made both within and between cohorts, due to potential for bias from committee members and even changing committee membership over time. Committees therefore need practical, defensible solutions to ensuring consistency and fairness.
One possible solution is the use of Bayesian Networks (BNs) as a pragmatic tool to inform programmatic deliberations and buttress committee decisions.
What is a Bayesian Network?
BNs are a flexible statistical model that can accommodate complex relationships between variables, and provide a visual and statistical framework for reasoning under uncertainty. They have been applied over several decades in disciplines including medicine, agriculture, finance, ecology and information technology, where they have fulfilled numerous functions including diagnosis, prediction, classification and decision-making.
BNs are widely used because they enable flexible and intuitive modelling of uncertainty and complexity in almost any real-world system where alternative statistical models prove inadequate. They are thus highly suitable for programmatic approaches that embrace the sampling of diverse evidence at different points in time to appraise the development of competence.
A BN consists of a number of ‘nodes’ that represent random variables. These nodes are connected by edges or arcs which are represented as arrows. Each node has an underlying conditional probability table or function that specifies how the probability of each possible state within that node (a so-called child node) depends on its parent nodes.
FIGURE 1: Possible BN for an overall progression decision relating to knowledge of three basic sciences domains
BNs provide not only a record of the evidentiary trail that led to final decisions; BNs can be fed with real data over time. Data from previous committee reviews can be fed in, allowing for probability calculations to emerge regarding the likelihood of Pass/Fail outcomes based on multiple decision-making points.
As observations are entered into a BN, a process referred to as ‘belief propagation’ occurs. In this process, the probabilities of other nodes in the network are updated to reflect the current belief about the most likely state of the system. For example, in Figure 1, the probability of progression for a trainee for whom we have no assessment information is 85 per cent; however, if the trainee were to fail one or more of the assessment components, the probability of progression would be reduced.
The BN is thus a form of an ‘expert system’ that recommends a final outcome. This does not imply that BNs should become a type of ‘black box’ or artificial intelligence machine that replaces the process of committee decision-making. Instead, we see the BN as another tool in the arsenal of evidence available to the assessment panel to add a layer of defensibility to the process.
Benefits for programmatic assessment
BNs enable committees to confirm their qualitative judgments against quantitative ‘recommendations’ from the probabilistic pathways that emerge from the BN. Using BNs as a tool where programmatic assessment is implemented also allows for the visual recording of committee decisions in context.
Programmatic committees must account for multiple, disparate attributes and observations with the additional challenge of properly capturing the nature of the relationships between these variables. Furthermore, there may be a requirement to impose certain policies that dictate hard rules or deterministic relationships between these variables – for example, if progression is conditional on passing a specific task. Fortunately, BNs provide considerable flexibility in these respects and can be used to ensure that the same constellation of evidence would lead to the same decision, either for different trainees or at different points in time.
An attractive feature of BNs is that the current belief about a trainee's proficiencies and attributes can be updated progressively and in real-time as new assessment information becomes available. Missing data or assessment evidence that has gaps (due to trainee rotations, for example) can be managed because BNs do not require complete data in order to support the probabilistic inference processes. BNs also afford the possibility of making inferences about the same collection of skills and attributes at separate points in time in a medical education program – effectively tracking learning progressions over time.
Implementation considerations
There is the matter that some medical education programs attract small candidatures, and therefore, the feasibility of BNs needs to be proven in programs that differ in size and other respects. Similarly, there is a need to explore the feasibility and robustness of BNs with different types of variables, at different levels of granularity, and incorporating observations that are of different levels of quality.
Although further research is required, BNs have the potential to aid programmatic assessment committees with managing the accumulation of evidence, supporting the process of making inferences under conditions of uncertainty, and in enhancing the robustness of a programmatic approach to assessment in medical education.
Overall, BNs have the potential to become a powerful tool to add a layer of defensibility to the process of decision-making in programmatic assessment. ■
Read the full article:
‘The potential use of Bayesian Networks to support committee decisions in programmatic assessment’ by Nathan Zoanetti and Jacob Pearce, Medical Education, November 2020.
Find out more:
ACER has been involved in numerous assessment review, evaluation, development and reform activities in medical education contexts in recent years, and is currently working with several colleges in an ongoing basis. For further information about ACER’s capabilities in medical assessment, contact highereducation@acer.org
Find out more:
Nathan Zoanetti is presenting at our fully online Research Conference 2021. Register now!